How to call made-up data?What should I call these growth rates?How to call “Inliers” and “Outliers” in FrenchRatio “observed-to-expected” - how do you call it?How to create synthetic mortality data set?What do you call a “redundant” parameter?What do you call models that are not invariant to predictor order?Raising average: organic approach vs outlierHow to call label encoding in multi-label case?Naming of mathematical elements in GMM?How would you call matrix of response variables?
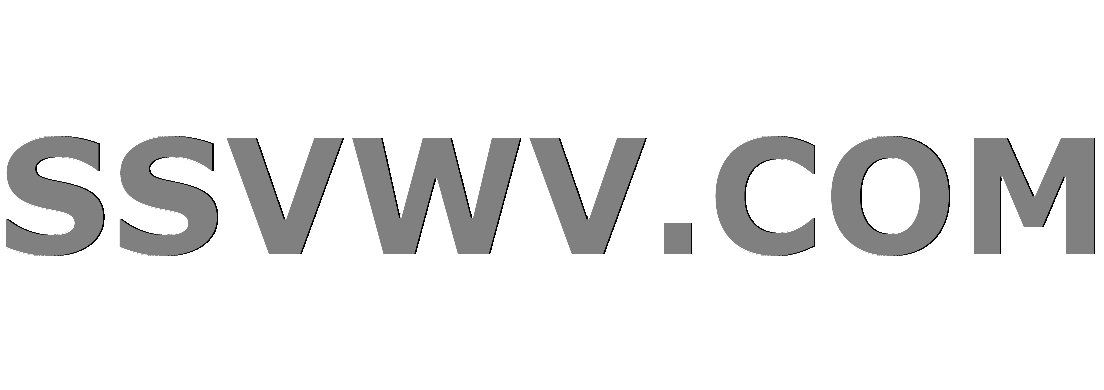
Multi tool use
How much can I judge a company based on a phone screening?
Why aren’t there water shutoff valves for each room?
What is the most difficult concept to grasp in Calculus 1?
Does an Irish VISA WARNING count as "refused entry at the border of any country other than the UK?"
Weird resistor with dots around it
Cusp forms have an orthonormal basis of eigenfunctions for all Hecke operators
Are there liquid fueled rocket boosters having coaxial fuel/oxidizer tanks?
List, map function based on a condition
Did Michelle Obama have a staff of 23; and Melania have a staff of 4?
What was the intention with the Commodore 128?
How can I find files in directories listed in a file?
Why does this Jet Provost strikemaster have a textured leading edge?
Did Pope Urban II issue the papal bull "terra nullius" in 1095?
What can I do to increase the amount of LEDs I can power with a pro micro?
What would it take to get a message to another star?
What is a "soap"?
What is the prop for Thor's hammer (Mjölnir) made of?
What is the farthest a camera can see?
Heyawake: An Introductory Puzzle
What if a restaurant suddenly cannot accept credit cards, and the customer has no cash?
How does the Moon's gravity affect Earth's oceans despite Earth's stronger gravitational pull?
What are the advantages of this gold finger shape?
How can I shoot a bow using Strength instead of Dexterity?
Is there a word for returning to unpreparedness?
How to call made-up data?
What should I call these growth rates?How to call “Inliers” and “Outliers” in FrenchRatio “observed-to-expected” - how do you call it?How to create synthetic mortality data set?What do you call a “redundant” parameter?What do you call models that are not invariant to predictor order?Raising average: organic approach vs outlierHow to call label encoding in multi-label case?Naming of mathematical elements in GMM?How would you call matrix of response variables?
.everyoneloves__top-leaderboard:empty,.everyoneloves__mid-leaderboard:empty,.everyoneloves__bot-mid-leaderboard:empty margin-bottom:0;
$begingroup$
I'm writing an example and have made up some data. I want it to be clear to the reader this is not real data, but I also don't want to give the impression of malice, since it just serves as an example.
There is no (pseudo)random component to this particular data, so it seems to me that 'simulated' is not appropriate. If I call it fictitious or fabricated, does that give the impression of fraudulent data? Is 'made-up' a word that would fit in a scientific context?
What is the terminology in statistical literature for non-simulated made-up data?
terminology synthetic-data
$endgroup$
add a comment |
$begingroup$
I'm writing an example and have made up some data. I want it to be clear to the reader this is not real data, but I also don't want to give the impression of malice, since it just serves as an example.
There is no (pseudo)random component to this particular data, so it seems to me that 'simulated' is not appropriate. If I call it fictitious or fabricated, does that give the impression of fraudulent data? Is 'made-up' a word that would fit in a scientific context?
What is the terminology in statistical literature for non-simulated made-up data?
terminology synthetic-data
$endgroup$
8
$begingroup$
Just to add a comment which spreads across several answer: "synthetic" is a good word for made up data which tries to look as realistic as possible, while "mock up" suggests data which has been crafted to demonstrate something particular. For example, "mock up" data might contain absurd outliers, just to demonstrate how important it is to deal with outliers properly.
$endgroup$
– Cort Ammon
Aug 4 at 20:02
add a comment |
$begingroup$
I'm writing an example and have made up some data. I want it to be clear to the reader this is not real data, but I also don't want to give the impression of malice, since it just serves as an example.
There is no (pseudo)random component to this particular data, so it seems to me that 'simulated' is not appropriate. If I call it fictitious or fabricated, does that give the impression of fraudulent data? Is 'made-up' a word that would fit in a scientific context?
What is the terminology in statistical literature for non-simulated made-up data?
terminology synthetic-data
$endgroup$
I'm writing an example and have made up some data. I want it to be clear to the reader this is not real data, but I also don't want to give the impression of malice, since it just serves as an example.
There is no (pseudo)random component to this particular data, so it seems to me that 'simulated' is not appropriate. If I call it fictitious or fabricated, does that give the impression of fraudulent data? Is 'made-up' a word that would fit in a scientific context?
What is the terminology in statistical literature for non-simulated made-up data?
terminology synthetic-data
terminology synthetic-data
edited Aug 4 at 9:17


kjetil b halvorsen
36.1k9 gold badges90 silver badges281 bronze badges
36.1k9 gold badges90 silver badges281 bronze badges
asked Aug 4 at 4:19
Frans RodenburgFrans Rodenburg
5,5611 gold badge8 silver badges33 bronze badges
5,5611 gold badge8 silver badges33 bronze badges
8
$begingroup$
Just to add a comment which spreads across several answer: "synthetic" is a good word for made up data which tries to look as realistic as possible, while "mock up" suggests data which has been crafted to demonstrate something particular. For example, "mock up" data might contain absurd outliers, just to demonstrate how important it is to deal with outliers properly.
$endgroup$
– Cort Ammon
Aug 4 at 20:02
add a comment |
8
$begingroup$
Just to add a comment which spreads across several answer: "synthetic" is a good word for made up data which tries to look as realistic as possible, while "mock up" suggests data which has been crafted to demonstrate something particular. For example, "mock up" data might contain absurd outliers, just to demonstrate how important it is to deal with outliers properly.
$endgroup$
– Cort Ammon
Aug 4 at 20:02
8
8
$begingroup$
Just to add a comment which spreads across several answer: "synthetic" is a good word for made up data which tries to look as realistic as possible, while "mock up" suggests data which has been crafted to demonstrate something particular. For example, "mock up" data might contain absurd outliers, just to demonstrate how important it is to deal with outliers properly.
$endgroup$
– Cort Ammon
Aug 4 at 20:02
$begingroup$
Just to add a comment which spreads across several answer: "synthetic" is a good word for made up data which tries to look as realistic as possible, while "mock up" suggests data which has been crafted to demonstrate something particular. For example, "mock up" data might contain absurd outliers, just to demonstrate how important it is to deal with outliers properly.
$endgroup$
– Cort Ammon
Aug 4 at 20:02
add a comment |
8 Answers
8
active
oldest
votes
$begingroup$
I would probably call this "synthetic" or "artificial" data, though I might also call it "simulated" (the simulation is just very simple).
$endgroup$
29
$begingroup$
One hears "toy data," "toy example," and "dummy data." Also I agree that "simulated" might well fit even in the absence of random numbers.
$endgroup$
– rolando2
Aug 4 at 9:33
7
$begingroup$
"Illustrative data" or "example data" might also work
$endgroup$
– Henry
Aug 4 at 9:44
8
$begingroup$
+1 'synthetic data' and 'toy example' are both terms I might use, if the occasion arose, as is 'constructed example'. Sometimes I say "illustrative example" or something similar, particularly when the example was explicitly constructed to have particular features (e.g. when designed as a counterexample to some mistaken notion).
$endgroup$
– Glen_b♦
Aug 4 at 10:10
1
$begingroup$
I tend to use toy data (without artificial or simulated) for real (measured) data sets that I "abuse" to demonstrate something.
$endgroup$
– cbeleites
Aug 5 at 17:27
1
$begingroup$
It depends a bit on your application what will work best. For example, I am also doing a project with "fake" data, but another part of the project involves using a computer model simulation. So it might confuse the reader for me to refer to the fake data as "simulated", falsely implying the data come from the simulation. So I've been relying on "artificial", and at times I describe the data as "manufactured". I personally would avoid "synthetic" as to me this term would imply that the data is some sort of combination of other data sources (a "synthesis" of e.g. data A and data B).
$endgroup$
– Ceph
Aug 6 at 20:26
|
show 1 more comment
$begingroup$
If you want to refer to your data as fictitious you'd be in good company, as that's the term Francis Anscombe used to describe his now famous quartet.
From Anscombe, F. J. (1973). "Graphs in Statistical Analysis", Am. Stat. 27 (1):
Some of these points are illustrated by four fictitious data sets,
each consisting of eleven (x, y) pairs, shown in the table.
But I think your caution is well placed, as my OED (v4) seems to indicates that this use of fictitious is obsolete
fictitious, a.
(fɪkˈtɪʃəs)
[f. L. fictīci-us (f. fingĕre to fashion, feign) + -ous: see -itious.]
1.1 †a.1.a Artificial as opposed to natural (obs.). b.1.b Counterfeit, ‘imitation’, sham; not genuine.
$endgroup$
$begingroup$
In terms of readability the first suggestion & the comments are much better alternative. No need to use uncommon, complicated words.
$endgroup$
– Tim♦
Aug 4 at 14:38
1
$begingroup$
@Tim: I want to agree, but I'm not entirely sure what I'd be agreeing with. Are you saying that fictitious would be a bad choice, despite having been used in a similar context before? Because that's what I'm saying.
$endgroup$
– AkselA
Aug 4 at 14:46
add a comment |
$begingroup$
In IT we often call it mockup data, which can presented through a mockup (application).
The mockup data can also be presented through a fully functional application, for instance to test the functionality of the application in a controlled manner.
$endgroup$
4
$begingroup$
Good point, but I believe that mockup data and simulated data are not exactly the same. When creating mockup data for unit tests, you need it only to preserve some very basic properties of the real data, while when using simulated data for statistical analysis, you usually use more sophisticated data examples.
$endgroup$
– Tim♦
Aug 4 at 20:02
2
$begingroup$
I still believe ErikE is correct though, when you write analytical code you either need the real thing or mock data. Mock data can be as big as you want it to be imo.
$endgroup$
– Mathijs Segers
Aug 6 at 5:52
1
$begingroup$
Practices probably vary as does use of terminology, I guess. For many of our tests and analyses we use live data which has been "defused" for reasons of security and anonymity. For others we create bare bones data just as Tim describes. I have no strong opinion but we do use the term mockup quite loosely.
$endgroup$
– ErikE
Aug 6 at 10:22
add a comment |
$begingroup$
I've seen repeated suggestions for the term "synthetic data". That term however has a broadly used, and very different meaning from what you want to express: https://en.wikipedia.org/wiki/Synthetic_data
I am not sure there is a generally accepted scientific term, but the term "example data" seems hard to misunderstand?
$endgroup$
1
$begingroup$
That article seems a little confused--the relationship to anonymization is pretty tenuous.
$endgroup$
– Matt Krause
Aug 6 at 16:29
$begingroup$
+1 but I agree with previous comment: apart from the second paragraphs (saying that synthesized data is a type of anonymized data), the rest of that Wikipedia article does seem to be describing what the questioner wants. I.e. realistic-looking made-up data.
$endgroup$
– Darren Cook
Aug 9 at 11:09
add a comment |
$begingroup$
I've encountered the term 'fake data' a fair amount. I guess it could have some negative connotations but I've heard it often enough that it doesn't register negatively at all for me.
FWIW, Andrew Gelman uses it too:
https://statmodeling.stat.columbia.edu/2009/09/04/fake-data_simul/
https://statmodeling.stat.columbia.edu/2019/03/23/yes-i-really-really-really-like-fake-data-simulation-and-i-cant-stop-talking-about-it/
https://books.google.dk/books?id=lV3DIdV0F9AC&pg=PA155&lpg=PA155&dq=fake+data+simulation&source=bl&ots=6ljKB6StQ4&sig=ACfU3U17GLP_84q_HfIQB4u5O6wV0yA2Aw&hl=en&sa=X&ved=2ahUKEwiF2_eB0uvjAhWswcQBHSn5Cn04ChDoATAAegQICRAB#v=onepage&q=fake%20data%20simulation&f=false
A quick google search for 'fake data' turns up a lot of results that seem to be using the term similarly:
https://scientistseessquirrel.wordpress.com/2016/03/10/good-uses-for-fake-data-part-1/
http://modernstatisticalworkflow.blogspot.com/2017/04/an-easy-way-to-simulate-fake-data-from.html
https://clayford.github.io/dwir/dwr_12_generating_data.html
And there's even a fakeR
package, which suggests that this is relatively common:
https://cran.r-project.org/web/packages/fakeR/fakeR.pdf
$endgroup$
add a comment |
$begingroup$
I use a different word depending on the manner in which I use the data. If I have found the made-up dataset lying around and have pointed my algorithm at it in a confirmatory manner, then the word "synthetic" is fine.
However, oftentimes whenever I use this type of data, I have invented the data with the specific intent of showing off the capabilities of my algorithm. In other words, I invented data for the specific purpose of getting "good results". In such circumstances, I am fond of the term "contrived" along with an explanation of my expectations for the data. This is because I don't want anyone to make the mistake of thinking that I pointed my algorithm at some arbitrary synthetic dataset I found lying around and it really worked out well. If I have cherry-picked data (to the point of actually making it up) specifically to make my algorithm work out well, I say so. This is because such results provide evidence that my algorithm can work out well, but provide only very weak evidence that one might expect the algorithm to work out well in general. The word "contrived" really sums up nicely the fact that I have chosen the data with "good results" in mind, a priori.
"does that give the impression of fraudulent data?"
No, but, it is important to be clear about the source of any dataset and your a priori expectations as the experimenter when reporting your results on any dataset. The term "fraud" explicitly includes an aspect of having covered something up or having outright lied. The #1 way to avoid commission of fraud in science is to simply be honest and forthright about the nature of your data and your expectations. In other words, if your data are fabricated and you fail to say as much in any way, and there is some kind of expectation that the data are not fabricated or, worse, you claim that the data are gathered in some non-fabricated sort of way, then that is "fraud". Don't do that thing. If you want to use some synonym for the term "fabricated" that "sounds better", such as "synthetic", nobody will fault you, but at the same time I don't think that anyone will notice the difference except for you.
A side note:
Less obvious are circumstances where one claims to have had a priori expectations that are actually post hoc explanations. This is also fraudulent analysis of data.
There is a danger of this when one chooses data specifically with the intent of "showing off" the capabilities of an algorithm, which is frequently the case with synthetic data.
To be clear about why this is the case, consider that the "normal" scientific method works something like so: 1) A population $D$ is chosen 2) A hypothesis $H$ is concieved 3) $H$ is tested against $D$ (or some sample chosen from $D$). Science doesn't have to work within this narrow definition, but this is what is called "confirmatory" analysis, and is generally considered the strongest form of evidence one can provide. Since the order of events correlates with the strength of evidence, it is important to specifically document them.
Notably, in the case of "contrived" data, the process often works more like so: 1) A hypothesis $H$ is conceived, 2) A population $D$ is chosen, 3) $H$ is tested against $D$. If you are testing an algorithm, for example, then the hypothesis that your fancy new algorithm "does a good job" might occur prior to the invention of the synthetic dataset. If this is the case, you should mention it. At the very least you should not purport that events transpired in a "confirmatory" manner, because that would lead readers to conclude that your evidence is stronger than it actually is.
There is no problem with doing this, so long as you are honest and forthright about what you have done. If you have gone through pains to create a dataset that gives "good results", do say so. As long as you let the reader know the steps that you have taken in your data analysis, they have the information necessary to effectively weigh the evidence for or against your hypotheses. When you are not honest or are not forthright, then this may give the impression that your evidence is stronger than it really is. When you are KNOWINGLY less than honest and forthright for the sake of making your evidence seem stronger than it really is, then that is, indeed, fraudulent.
In any case, this is why I prefer the term "contrived" for such datasets, along with a short explanation that they are, indeed, chosen with a hypothesis in mind. "Contrived" conveys the sense that not only did I create a synthetic dataset, but I did so with particular intentions that reflect the fact that my hypothesis was already in place before the creation of my dataset.
To illustrate by an example: You create an algorithm for analysis of arbitrary time-series. You hypothesize that this algorithm will give "good results" when pointed at time-series. Consider, now, the following two possibilities:
1) You create some synthetic data that looks like the sort of thing that you expect your algorithm to perform well on. You analyze this data and the algorithm performs well. 2) You grab some synthetic datasets because they are available why not. You analyze this data and the algorithm performs well. Which of these two circumstances provides the better evidence that your algorithm performs well on arbitrary time-series? Clearly, it is option 2. However, it might be easy to report in either option 1 or option 2 that "we applied algorithm $A$ to synthetic dataset $D$. Results are shown in Figure $x.y$." In the absence of any context, a reader might reasonably assume that these results are confirmatory (option 2), when, in the case of option 1, they are not. The reader has therefore, in option 1, been given the impression that the evidence is stronger than it really is.
tl;dr
Use whatever term you like, "synthetic", "contrived", "fabricated", "fictitious". However, the term that you use is insufficient to ensure that your results are not misleading. Ensure that you are clear in your report about how the data came about, including your expectations for the data and the reasons why you chose the data that you chose.
$endgroup$
add a comment |
$begingroup$
Intuitively I would go to the term 'Dummy data', in the same sense that "Lorem ipsum..." is called 'Dummy text'.
The word 'Dummy' is quite general and easy to understand for people from various backgrounds and is therfore less likely to be misinterpreted by readers of a less statistical background.
$endgroup$
2
$begingroup$
If it's in a regression context, I would avoid overloading "dummy", lest you have dummy variables encoding dummy data.
$endgroup$
– Matt Krause
Aug 5 at 21:24
add a comment |
$begingroup$
In business you would call it synthetic data predominantly - and occasionally simulated data or fake data. In academia you would call it pseudo-data predominantly, and occasionally simulated data. If it is the result of a Monte Carlo simulation, at times it gets referred colloquially as simply “Monte Carlo”.
$endgroup$
2
$begingroup$
"Monte Carlo" is the name of the method, so the "colloquial" name would be very misleading.
$endgroup$
– Tim♦
Aug 5 at 21:30
$begingroup$
It’s jargon - and as any other technical jargon is meant to create a community around it, by leaving the rest of the world wondering what the hell is this about. So yes it is used and yes it is (quite voluntarily) misleading
$endgroup$
– famargar
Aug 5 at 21:38
add a comment |
Your Answer
StackExchange.ready(function()
var channelOptions =
tags: "".split(" "),
id: "65"
;
initTagRenderer("".split(" "), "".split(" "), channelOptions);
StackExchange.using("externalEditor", function()
// Have to fire editor after snippets, if snippets enabled
if (StackExchange.settings.snippets.snippetsEnabled)
StackExchange.using("snippets", function()
createEditor();
);
else
createEditor();
);
function createEditor()
StackExchange.prepareEditor(
heartbeatType: 'answer',
autoActivateHeartbeat: false,
convertImagesToLinks: false,
noModals: true,
showLowRepImageUploadWarning: true,
reputationToPostImages: null,
bindNavPrevention: true,
postfix: "",
imageUploader:
brandingHtml: "Powered by u003ca class="icon-imgur-white" href="https://imgur.com/"u003eu003c/au003e",
contentPolicyHtml: "User contributions licensed under u003ca href="https://creativecommons.org/licenses/by-sa/3.0/"u003ecc by-sa 3.0 with attribution requiredu003c/au003e u003ca href="https://stackoverflow.com/legal/content-policy"u003e(content policy)u003c/au003e",
allowUrls: true
,
onDemand: true,
discardSelector: ".discard-answer"
,immediatelyShowMarkdownHelp:true
);
);
Sign up or log in
StackExchange.ready(function ()
StackExchange.helpers.onClickDraftSave('#login-link');
);
Sign up using Google
Sign up using Facebook
Sign up using Email and Password
Post as a guest
Required, but never shown
StackExchange.ready(
function ()
StackExchange.openid.initPostLogin('.new-post-login', 'https%3a%2f%2fstats.stackexchange.com%2fquestions%2f420525%2fhow-to-call-made-up-data%23new-answer', 'question_page');
);
Post as a guest
Required, but never shown
8 Answers
8
active
oldest
votes
8 Answers
8
active
oldest
votes
active
oldest
votes
active
oldest
votes
$begingroup$
I would probably call this "synthetic" or "artificial" data, though I might also call it "simulated" (the simulation is just very simple).
$endgroup$
29
$begingroup$
One hears "toy data," "toy example," and "dummy data." Also I agree that "simulated" might well fit even in the absence of random numbers.
$endgroup$
– rolando2
Aug 4 at 9:33
7
$begingroup$
"Illustrative data" or "example data" might also work
$endgroup$
– Henry
Aug 4 at 9:44
8
$begingroup$
+1 'synthetic data' and 'toy example' are both terms I might use, if the occasion arose, as is 'constructed example'. Sometimes I say "illustrative example" or something similar, particularly when the example was explicitly constructed to have particular features (e.g. when designed as a counterexample to some mistaken notion).
$endgroup$
– Glen_b♦
Aug 4 at 10:10
1
$begingroup$
I tend to use toy data (without artificial or simulated) for real (measured) data sets that I "abuse" to demonstrate something.
$endgroup$
– cbeleites
Aug 5 at 17:27
1
$begingroup$
It depends a bit on your application what will work best. For example, I am also doing a project with "fake" data, but another part of the project involves using a computer model simulation. So it might confuse the reader for me to refer to the fake data as "simulated", falsely implying the data come from the simulation. So I've been relying on "artificial", and at times I describe the data as "manufactured". I personally would avoid "synthetic" as to me this term would imply that the data is some sort of combination of other data sources (a "synthesis" of e.g. data A and data B).
$endgroup$
– Ceph
Aug 6 at 20:26
|
show 1 more comment
$begingroup$
I would probably call this "synthetic" or "artificial" data, though I might also call it "simulated" (the simulation is just very simple).
$endgroup$
29
$begingroup$
One hears "toy data," "toy example," and "dummy data." Also I agree that "simulated" might well fit even in the absence of random numbers.
$endgroup$
– rolando2
Aug 4 at 9:33
7
$begingroup$
"Illustrative data" or "example data" might also work
$endgroup$
– Henry
Aug 4 at 9:44
8
$begingroup$
+1 'synthetic data' and 'toy example' are both terms I might use, if the occasion arose, as is 'constructed example'. Sometimes I say "illustrative example" or something similar, particularly when the example was explicitly constructed to have particular features (e.g. when designed as a counterexample to some mistaken notion).
$endgroup$
– Glen_b♦
Aug 4 at 10:10
1
$begingroup$
I tend to use toy data (without artificial or simulated) for real (measured) data sets that I "abuse" to demonstrate something.
$endgroup$
– cbeleites
Aug 5 at 17:27
1
$begingroup$
It depends a bit on your application what will work best. For example, I am also doing a project with "fake" data, but another part of the project involves using a computer model simulation. So it might confuse the reader for me to refer to the fake data as "simulated", falsely implying the data come from the simulation. So I've been relying on "artificial", and at times I describe the data as "manufactured". I personally would avoid "synthetic" as to me this term would imply that the data is some sort of combination of other data sources (a "synthesis" of e.g. data A and data B).
$endgroup$
– Ceph
Aug 6 at 20:26
|
show 1 more comment
$begingroup$
I would probably call this "synthetic" or "artificial" data, though I might also call it "simulated" (the simulation is just very simple).
$endgroup$
I would probably call this "synthetic" or "artificial" data, though I might also call it "simulated" (the simulation is just very simple).
answered Aug 4 at 4:38
Louis CialdellaLouis Cialdella
1,0428 silver badges13 bronze badges
1,0428 silver badges13 bronze badges
29
$begingroup$
One hears "toy data," "toy example," and "dummy data." Also I agree that "simulated" might well fit even in the absence of random numbers.
$endgroup$
– rolando2
Aug 4 at 9:33
7
$begingroup$
"Illustrative data" or "example data" might also work
$endgroup$
– Henry
Aug 4 at 9:44
8
$begingroup$
+1 'synthetic data' and 'toy example' are both terms I might use, if the occasion arose, as is 'constructed example'. Sometimes I say "illustrative example" or something similar, particularly when the example was explicitly constructed to have particular features (e.g. when designed as a counterexample to some mistaken notion).
$endgroup$
– Glen_b♦
Aug 4 at 10:10
1
$begingroup$
I tend to use toy data (without artificial or simulated) for real (measured) data sets that I "abuse" to demonstrate something.
$endgroup$
– cbeleites
Aug 5 at 17:27
1
$begingroup$
It depends a bit on your application what will work best. For example, I am also doing a project with "fake" data, but another part of the project involves using a computer model simulation. So it might confuse the reader for me to refer to the fake data as "simulated", falsely implying the data come from the simulation. So I've been relying on "artificial", and at times I describe the data as "manufactured". I personally would avoid "synthetic" as to me this term would imply that the data is some sort of combination of other data sources (a "synthesis" of e.g. data A and data B).
$endgroup$
– Ceph
Aug 6 at 20:26
|
show 1 more comment
29
$begingroup$
One hears "toy data," "toy example," and "dummy data." Also I agree that "simulated" might well fit even in the absence of random numbers.
$endgroup$
– rolando2
Aug 4 at 9:33
7
$begingroup$
"Illustrative data" or "example data" might also work
$endgroup$
– Henry
Aug 4 at 9:44
8
$begingroup$
+1 'synthetic data' and 'toy example' are both terms I might use, if the occasion arose, as is 'constructed example'. Sometimes I say "illustrative example" or something similar, particularly when the example was explicitly constructed to have particular features (e.g. when designed as a counterexample to some mistaken notion).
$endgroup$
– Glen_b♦
Aug 4 at 10:10
1
$begingroup$
I tend to use toy data (without artificial or simulated) for real (measured) data sets that I "abuse" to demonstrate something.
$endgroup$
– cbeleites
Aug 5 at 17:27
1
$begingroup$
It depends a bit on your application what will work best. For example, I am also doing a project with "fake" data, but another part of the project involves using a computer model simulation. So it might confuse the reader for me to refer to the fake data as "simulated", falsely implying the data come from the simulation. So I've been relying on "artificial", and at times I describe the data as "manufactured". I personally would avoid "synthetic" as to me this term would imply that the data is some sort of combination of other data sources (a "synthesis" of e.g. data A and data B).
$endgroup$
– Ceph
Aug 6 at 20:26
29
29
$begingroup$
One hears "toy data," "toy example," and "dummy data." Also I agree that "simulated" might well fit even in the absence of random numbers.
$endgroup$
– rolando2
Aug 4 at 9:33
$begingroup$
One hears "toy data," "toy example," and "dummy data." Also I agree that "simulated" might well fit even in the absence of random numbers.
$endgroup$
– rolando2
Aug 4 at 9:33
7
7
$begingroup$
"Illustrative data" or "example data" might also work
$endgroup$
– Henry
Aug 4 at 9:44
$begingroup$
"Illustrative data" or "example data" might also work
$endgroup$
– Henry
Aug 4 at 9:44
8
8
$begingroup$
+1 'synthetic data' and 'toy example' are both terms I might use, if the occasion arose, as is 'constructed example'. Sometimes I say "illustrative example" or something similar, particularly when the example was explicitly constructed to have particular features (e.g. when designed as a counterexample to some mistaken notion).
$endgroup$
– Glen_b♦
Aug 4 at 10:10
$begingroup$
+1 'synthetic data' and 'toy example' are both terms I might use, if the occasion arose, as is 'constructed example'. Sometimes I say "illustrative example" or something similar, particularly when the example was explicitly constructed to have particular features (e.g. when designed as a counterexample to some mistaken notion).
$endgroup$
– Glen_b♦
Aug 4 at 10:10
1
1
$begingroup$
I tend to use toy data (without artificial or simulated) for real (measured) data sets that I "abuse" to demonstrate something.
$endgroup$
– cbeleites
Aug 5 at 17:27
$begingroup$
I tend to use toy data (without artificial or simulated) for real (measured) data sets that I "abuse" to demonstrate something.
$endgroup$
– cbeleites
Aug 5 at 17:27
1
1
$begingroup$
It depends a bit on your application what will work best. For example, I am also doing a project with "fake" data, but another part of the project involves using a computer model simulation. So it might confuse the reader for me to refer to the fake data as "simulated", falsely implying the data come from the simulation. So I've been relying on "artificial", and at times I describe the data as "manufactured". I personally would avoid "synthetic" as to me this term would imply that the data is some sort of combination of other data sources (a "synthesis" of e.g. data A and data B).
$endgroup$
– Ceph
Aug 6 at 20:26
$begingroup$
It depends a bit on your application what will work best. For example, I am also doing a project with "fake" data, but another part of the project involves using a computer model simulation. So it might confuse the reader for me to refer to the fake data as "simulated", falsely implying the data come from the simulation. So I've been relying on "artificial", and at times I describe the data as "manufactured". I personally would avoid "synthetic" as to me this term would imply that the data is some sort of combination of other data sources (a "synthesis" of e.g. data A and data B).
$endgroup$
– Ceph
Aug 6 at 20:26
|
show 1 more comment
$begingroup$
If you want to refer to your data as fictitious you'd be in good company, as that's the term Francis Anscombe used to describe his now famous quartet.
From Anscombe, F. J. (1973). "Graphs in Statistical Analysis", Am. Stat. 27 (1):
Some of these points are illustrated by four fictitious data sets,
each consisting of eleven (x, y) pairs, shown in the table.
But I think your caution is well placed, as my OED (v4) seems to indicates that this use of fictitious is obsolete
fictitious, a.
(fɪkˈtɪʃəs)
[f. L. fictīci-us (f. fingĕre to fashion, feign) + -ous: see -itious.]
1.1 †a.1.a Artificial as opposed to natural (obs.). b.1.b Counterfeit, ‘imitation’, sham; not genuine.
$endgroup$
$begingroup$
In terms of readability the first suggestion & the comments are much better alternative. No need to use uncommon, complicated words.
$endgroup$
– Tim♦
Aug 4 at 14:38
1
$begingroup$
@Tim: I want to agree, but I'm not entirely sure what I'd be agreeing with. Are you saying that fictitious would be a bad choice, despite having been used in a similar context before? Because that's what I'm saying.
$endgroup$
– AkselA
Aug 4 at 14:46
add a comment |
$begingroup$
If you want to refer to your data as fictitious you'd be in good company, as that's the term Francis Anscombe used to describe his now famous quartet.
From Anscombe, F. J. (1973). "Graphs in Statistical Analysis", Am. Stat. 27 (1):
Some of these points are illustrated by four fictitious data sets,
each consisting of eleven (x, y) pairs, shown in the table.
But I think your caution is well placed, as my OED (v4) seems to indicates that this use of fictitious is obsolete
fictitious, a.
(fɪkˈtɪʃəs)
[f. L. fictīci-us (f. fingĕre to fashion, feign) + -ous: see -itious.]
1.1 †a.1.a Artificial as opposed to natural (obs.). b.1.b Counterfeit, ‘imitation’, sham; not genuine.
$endgroup$
$begingroup$
In terms of readability the first suggestion & the comments are much better alternative. No need to use uncommon, complicated words.
$endgroup$
– Tim♦
Aug 4 at 14:38
1
$begingroup$
@Tim: I want to agree, but I'm not entirely sure what I'd be agreeing with. Are you saying that fictitious would be a bad choice, despite having been used in a similar context before? Because that's what I'm saying.
$endgroup$
– AkselA
Aug 4 at 14:46
add a comment |
$begingroup$
If you want to refer to your data as fictitious you'd be in good company, as that's the term Francis Anscombe used to describe his now famous quartet.
From Anscombe, F. J. (1973). "Graphs in Statistical Analysis", Am. Stat. 27 (1):
Some of these points are illustrated by four fictitious data sets,
each consisting of eleven (x, y) pairs, shown in the table.
But I think your caution is well placed, as my OED (v4) seems to indicates that this use of fictitious is obsolete
fictitious, a.
(fɪkˈtɪʃəs)
[f. L. fictīci-us (f. fingĕre to fashion, feign) + -ous: see -itious.]
1.1 †a.1.a Artificial as opposed to natural (obs.). b.1.b Counterfeit, ‘imitation’, sham; not genuine.
$endgroup$
If you want to refer to your data as fictitious you'd be in good company, as that's the term Francis Anscombe used to describe his now famous quartet.
From Anscombe, F. J. (1973). "Graphs in Statistical Analysis", Am. Stat. 27 (1):
Some of these points are illustrated by four fictitious data sets,
each consisting of eleven (x, y) pairs, shown in the table.
But I think your caution is well placed, as my OED (v4) seems to indicates that this use of fictitious is obsolete
fictitious, a.
(fɪkˈtɪʃəs)
[f. L. fictīci-us (f. fingĕre to fashion, feign) + -ous: see -itious.]
1.1 †a.1.a Artificial as opposed to natural (obs.). b.1.b Counterfeit, ‘imitation’, sham; not genuine.
edited Aug 4 at 22:53
answered Aug 4 at 14:26


AkselAAkselA
2811 silver badge12 bronze badges
2811 silver badge12 bronze badges
$begingroup$
In terms of readability the first suggestion & the comments are much better alternative. No need to use uncommon, complicated words.
$endgroup$
– Tim♦
Aug 4 at 14:38
1
$begingroup$
@Tim: I want to agree, but I'm not entirely sure what I'd be agreeing with. Are you saying that fictitious would be a bad choice, despite having been used in a similar context before? Because that's what I'm saying.
$endgroup$
– AkselA
Aug 4 at 14:46
add a comment |
$begingroup$
In terms of readability the first suggestion & the comments are much better alternative. No need to use uncommon, complicated words.
$endgroup$
– Tim♦
Aug 4 at 14:38
1
$begingroup$
@Tim: I want to agree, but I'm not entirely sure what I'd be agreeing with. Are you saying that fictitious would be a bad choice, despite having been used in a similar context before? Because that's what I'm saying.
$endgroup$
– AkselA
Aug 4 at 14:46
$begingroup$
In terms of readability the first suggestion & the comments are much better alternative. No need to use uncommon, complicated words.
$endgroup$
– Tim♦
Aug 4 at 14:38
$begingroup$
In terms of readability the first suggestion & the comments are much better alternative. No need to use uncommon, complicated words.
$endgroup$
– Tim♦
Aug 4 at 14:38
1
1
$begingroup$
@Tim: I want to agree, but I'm not entirely sure what I'd be agreeing with. Are you saying that fictitious would be a bad choice, despite having been used in a similar context before? Because that's what I'm saying.
$endgroup$
– AkselA
Aug 4 at 14:46
$begingroup$
@Tim: I want to agree, but I'm not entirely sure what I'd be agreeing with. Are you saying that fictitious would be a bad choice, despite having been used in a similar context before? Because that's what I'm saying.
$endgroup$
– AkselA
Aug 4 at 14:46
add a comment |
$begingroup$
In IT we often call it mockup data, which can presented through a mockup (application).
The mockup data can also be presented through a fully functional application, for instance to test the functionality of the application in a controlled manner.
$endgroup$
4
$begingroup$
Good point, but I believe that mockup data and simulated data are not exactly the same. When creating mockup data for unit tests, you need it only to preserve some very basic properties of the real data, while when using simulated data for statistical analysis, you usually use more sophisticated data examples.
$endgroup$
– Tim♦
Aug 4 at 20:02
2
$begingroup$
I still believe ErikE is correct though, when you write analytical code you either need the real thing or mock data. Mock data can be as big as you want it to be imo.
$endgroup$
– Mathijs Segers
Aug 6 at 5:52
1
$begingroup$
Practices probably vary as does use of terminology, I guess. For many of our tests and analyses we use live data which has been "defused" for reasons of security and anonymity. For others we create bare bones data just as Tim describes. I have no strong opinion but we do use the term mockup quite loosely.
$endgroup$
– ErikE
Aug 6 at 10:22
add a comment |
$begingroup$
In IT we often call it mockup data, which can presented through a mockup (application).
The mockup data can also be presented through a fully functional application, for instance to test the functionality of the application in a controlled manner.
$endgroup$
4
$begingroup$
Good point, but I believe that mockup data and simulated data are not exactly the same. When creating mockup data for unit tests, you need it only to preserve some very basic properties of the real data, while when using simulated data for statistical analysis, you usually use more sophisticated data examples.
$endgroup$
– Tim♦
Aug 4 at 20:02
2
$begingroup$
I still believe ErikE is correct though, when you write analytical code you either need the real thing or mock data. Mock data can be as big as you want it to be imo.
$endgroup$
– Mathijs Segers
Aug 6 at 5:52
1
$begingroup$
Practices probably vary as does use of terminology, I guess. For many of our tests and analyses we use live data which has been "defused" for reasons of security and anonymity. For others we create bare bones data just as Tim describes. I have no strong opinion but we do use the term mockup quite loosely.
$endgroup$
– ErikE
Aug 6 at 10:22
add a comment |
$begingroup$
In IT we often call it mockup data, which can presented through a mockup (application).
The mockup data can also be presented through a fully functional application, for instance to test the functionality of the application in a controlled manner.
$endgroup$
In IT we often call it mockup data, which can presented through a mockup (application).
The mockup data can also be presented through a fully functional application, for instance to test the functionality of the application in a controlled manner.
answered Aug 4 at 19:55
ErikEErikE
1792 bronze badges
1792 bronze badges
4
$begingroup$
Good point, but I believe that mockup data and simulated data are not exactly the same. When creating mockup data for unit tests, you need it only to preserve some very basic properties of the real data, while when using simulated data for statistical analysis, you usually use more sophisticated data examples.
$endgroup$
– Tim♦
Aug 4 at 20:02
2
$begingroup$
I still believe ErikE is correct though, when you write analytical code you either need the real thing or mock data. Mock data can be as big as you want it to be imo.
$endgroup$
– Mathijs Segers
Aug 6 at 5:52
1
$begingroup$
Practices probably vary as does use of terminology, I guess. For many of our tests and analyses we use live data which has been "defused" for reasons of security and anonymity. For others we create bare bones data just as Tim describes. I have no strong opinion but we do use the term mockup quite loosely.
$endgroup$
– ErikE
Aug 6 at 10:22
add a comment |
4
$begingroup$
Good point, but I believe that mockup data and simulated data are not exactly the same. When creating mockup data for unit tests, you need it only to preserve some very basic properties of the real data, while when using simulated data for statistical analysis, you usually use more sophisticated data examples.
$endgroup$
– Tim♦
Aug 4 at 20:02
2
$begingroup$
I still believe ErikE is correct though, when you write analytical code you either need the real thing or mock data. Mock data can be as big as you want it to be imo.
$endgroup$
– Mathijs Segers
Aug 6 at 5:52
1
$begingroup$
Practices probably vary as does use of terminology, I guess. For many of our tests and analyses we use live data which has been "defused" for reasons of security and anonymity. For others we create bare bones data just as Tim describes. I have no strong opinion but we do use the term mockup quite loosely.
$endgroup$
– ErikE
Aug 6 at 10:22
4
4
$begingroup$
Good point, but I believe that mockup data and simulated data are not exactly the same. When creating mockup data for unit tests, you need it only to preserve some very basic properties of the real data, while when using simulated data for statistical analysis, you usually use more sophisticated data examples.
$endgroup$
– Tim♦
Aug 4 at 20:02
$begingroup$
Good point, but I believe that mockup data and simulated data are not exactly the same. When creating mockup data for unit tests, you need it only to preserve some very basic properties of the real data, while when using simulated data for statistical analysis, you usually use more sophisticated data examples.
$endgroup$
– Tim♦
Aug 4 at 20:02
2
2
$begingroup$
I still believe ErikE is correct though, when you write analytical code you either need the real thing or mock data. Mock data can be as big as you want it to be imo.
$endgroup$
– Mathijs Segers
Aug 6 at 5:52
$begingroup$
I still believe ErikE is correct though, when you write analytical code you either need the real thing or mock data. Mock data can be as big as you want it to be imo.
$endgroup$
– Mathijs Segers
Aug 6 at 5:52
1
1
$begingroup$
Practices probably vary as does use of terminology, I guess. For many of our tests and analyses we use live data which has been "defused" for reasons of security and anonymity. For others we create bare bones data just as Tim describes. I have no strong opinion but we do use the term mockup quite loosely.
$endgroup$
– ErikE
Aug 6 at 10:22
$begingroup$
Practices probably vary as does use of terminology, I guess. For many of our tests and analyses we use live data which has been "defused" for reasons of security and anonymity. For others we create bare bones data just as Tim describes. I have no strong opinion but we do use the term mockup quite loosely.
$endgroup$
– ErikE
Aug 6 at 10:22
add a comment |
$begingroup$
I've seen repeated suggestions for the term "synthetic data". That term however has a broadly used, and very different meaning from what you want to express: https://en.wikipedia.org/wiki/Synthetic_data
I am not sure there is a generally accepted scientific term, but the term "example data" seems hard to misunderstand?
$endgroup$
1
$begingroup$
That article seems a little confused--the relationship to anonymization is pretty tenuous.
$endgroup$
– Matt Krause
Aug 6 at 16:29
$begingroup$
+1 but I agree with previous comment: apart from the second paragraphs (saying that synthesized data is a type of anonymized data), the rest of that Wikipedia article does seem to be describing what the questioner wants. I.e. realistic-looking made-up data.
$endgroup$
– Darren Cook
Aug 9 at 11:09
add a comment |
$begingroup$
I've seen repeated suggestions for the term "synthetic data". That term however has a broadly used, and very different meaning from what you want to express: https://en.wikipedia.org/wiki/Synthetic_data
I am not sure there is a generally accepted scientific term, but the term "example data" seems hard to misunderstand?
$endgroup$
1
$begingroup$
That article seems a little confused--the relationship to anonymization is pretty tenuous.
$endgroup$
– Matt Krause
Aug 6 at 16:29
$begingroup$
+1 but I agree with previous comment: apart from the second paragraphs (saying that synthesized data is a type of anonymized data), the rest of that Wikipedia article does seem to be describing what the questioner wants. I.e. realistic-looking made-up data.
$endgroup$
– Darren Cook
Aug 9 at 11:09
add a comment |
$begingroup$
I've seen repeated suggestions for the term "synthetic data". That term however has a broadly used, and very different meaning from what you want to express: https://en.wikipedia.org/wiki/Synthetic_data
I am not sure there is a generally accepted scientific term, but the term "example data" seems hard to misunderstand?
$endgroup$
I've seen repeated suggestions for the term "synthetic data". That term however has a broadly used, and very different meaning from what you want to express: https://en.wikipedia.org/wiki/Synthetic_data
I am not sure there is a generally accepted scientific term, but the term "example data" seems hard to misunderstand?
answered Aug 5 at 11:10
srasssrass
212 bronze badges
212 bronze badges
1
$begingroup$
That article seems a little confused--the relationship to anonymization is pretty tenuous.
$endgroup$
– Matt Krause
Aug 6 at 16:29
$begingroup$
+1 but I agree with previous comment: apart from the second paragraphs (saying that synthesized data is a type of anonymized data), the rest of that Wikipedia article does seem to be describing what the questioner wants. I.e. realistic-looking made-up data.
$endgroup$
– Darren Cook
Aug 9 at 11:09
add a comment |
1
$begingroup$
That article seems a little confused--the relationship to anonymization is pretty tenuous.
$endgroup$
– Matt Krause
Aug 6 at 16:29
$begingroup$
+1 but I agree with previous comment: apart from the second paragraphs (saying that synthesized data is a type of anonymized data), the rest of that Wikipedia article does seem to be describing what the questioner wants. I.e. realistic-looking made-up data.
$endgroup$
– Darren Cook
Aug 9 at 11:09
1
1
$begingroup$
That article seems a little confused--the relationship to anonymization is pretty tenuous.
$endgroup$
– Matt Krause
Aug 6 at 16:29
$begingroup$
That article seems a little confused--the relationship to anonymization is pretty tenuous.
$endgroup$
– Matt Krause
Aug 6 at 16:29
$begingroup$
+1 but I agree with previous comment: apart from the second paragraphs (saying that synthesized data is a type of anonymized data), the rest of that Wikipedia article does seem to be describing what the questioner wants. I.e. realistic-looking made-up data.
$endgroup$
– Darren Cook
Aug 9 at 11:09
$begingroup$
+1 but I agree with previous comment: apart from the second paragraphs (saying that synthesized data is a type of anonymized data), the rest of that Wikipedia article does seem to be describing what the questioner wants. I.e. realistic-looking made-up data.
$endgroup$
– Darren Cook
Aug 9 at 11:09
add a comment |
$begingroup$
I've encountered the term 'fake data' a fair amount. I guess it could have some negative connotations but I've heard it often enough that it doesn't register negatively at all for me.
FWIW, Andrew Gelman uses it too:
https://statmodeling.stat.columbia.edu/2009/09/04/fake-data_simul/
https://statmodeling.stat.columbia.edu/2019/03/23/yes-i-really-really-really-like-fake-data-simulation-and-i-cant-stop-talking-about-it/
https://books.google.dk/books?id=lV3DIdV0F9AC&pg=PA155&lpg=PA155&dq=fake+data+simulation&source=bl&ots=6ljKB6StQ4&sig=ACfU3U17GLP_84q_HfIQB4u5O6wV0yA2Aw&hl=en&sa=X&ved=2ahUKEwiF2_eB0uvjAhWswcQBHSn5Cn04ChDoATAAegQICRAB#v=onepage&q=fake%20data%20simulation&f=false
A quick google search for 'fake data' turns up a lot of results that seem to be using the term similarly:
https://scientistseessquirrel.wordpress.com/2016/03/10/good-uses-for-fake-data-part-1/
http://modernstatisticalworkflow.blogspot.com/2017/04/an-easy-way-to-simulate-fake-data-from.html
https://clayford.github.io/dwir/dwr_12_generating_data.html
And there's even a fakeR
package, which suggests that this is relatively common:
https://cran.r-project.org/web/packages/fakeR/fakeR.pdf
$endgroup$
add a comment |
$begingroup$
I've encountered the term 'fake data' a fair amount. I guess it could have some negative connotations but I've heard it often enough that it doesn't register negatively at all for me.
FWIW, Andrew Gelman uses it too:
https://statmodeling.stat.columbia.edu/2009/09/04/fake-data_simul/
https://statmodeling.stat.columbia.edu/2019/03/23/yes-i-really-really-really-like-fake-data-simulation-and-i-cant-stop-talking-about-it/
https://books.google.dk/books?id=lV3DIdV0F9AC&pg=PA155&lpg=PA155&dq=fake+data+simulation&source=bl&ots=6ljKB6StQ4&sig=ACfU3U17GLP_84q_HfIQB4u5O6wV0yA2Aw&hl=en&sa=X&ved=2ahUKEwiF2_eB0uvjAhWswcQBHSn5Cn04ChDoATAAegQICRAB#v=onepage&q=fake%20data%20simulation&f=false
A quick google search for 'fake data' turns up a lot of results that seem to be using the term similarly:
https://scientistseessquirrel.wordpress.com/2016/03/10/good-uses-for-fake-data-part-1/
http://modernstatisticalworkflow.blogspot.com/2017/04/an-easy-way-to-simulate-fake-data-from.html
https://clayford.github.io/dwir/dwr_12_generating_data.html
And there's even a fakeR
package, which suggests that this is relatively common:
https://cran.r-project.org/web/packages/fakeR/fakeR.pdf
$endgroup$
add a comment |
$begingroup$
I've encountered the term 'fake data' a fair amount. I guess it could have some negative connotations but I've heard it often enough that it doesn't register negatively at all for me.
FWIW, Andrew Gelman uses it too:
https://statmodeling.stat.columbia.edu/2009/09/04/fake-data_simul/
https://statmodeling.stat.columbia.edu/2019/03/23/yes-i-really-really-really-like-fake-data-simulation-and-i-cant-stop-talking-about-it/
https://books.google.dk/books?id=lV3DIdV0F9AC&pg=PA155&lpg=PA155&dq=fake+data+simulation&source=bl&ots=6ljKB6StQ4&sig=ACfU3U17GLP_84q_HfIQB4u5O6wV0yA2Aw&hl=en&sa=X&ved=2ahUKEwiF2_eB0uvjAhWswcQBHSn5Cn04ChDoATAAegQICRAB#v=onepage&q=fake%20data%20simulation&f=false
A quick google search for 'fake data' turns up a lot of results that seem to be using the term similarly:
https://scientistseessquirrel.wordpress.com/2016/03/10/good-uses-for-fake-data-part-1/
http://modernstatisticalworkflow.blogspot.com/2017/04/an-easy-way-to-simulate-fake-data-from.html
https://clayford.github.io/dwir/dwr_12_generating_data.html
And there's even a fakeR
package, which suggests that this is relatively common:
https://cran.r-project.org/web/packages/fakeR/fakeR.pdf
$endgroup$
I've encountered the term 'fake data' a fair amount. I guess it could have some negative connotations but I've heard it often enough that it doesn't register negatively at all for me.
FWIW, Andrew Gelman uses it too:
https://statmodeling.stat.columbia.edu/2009/09/04/fake-data_simul/
https://statmodeling.stat.columbia.edu/2019/03/23/yes-i-really-really-really-like-fake-data-simulation-and-i-cant-stop-talking-about-it/
https://books.google.dk/books?id=lV3DIdV0F9AC&pg=PA155&lpg=PA155&dq=fake+data+simulation&source=bl&ots=6ljKB6StQ4&sig=ACfU3U17GLP_84q_HfIQB4u5O6wV0yA2Aw&hl=en&sa=X&ved=2ahUKEwiF2_eB0uvjAhWswcQBHSn5Cn04ChDoATAAegQICRAB#v=onepage&q=fake%20data%20simulation&f=false
A quick google search for 'fake data' turns up a lot of results that seem to be using the term similarly:
https://scientistseessquirrel.wordpress.com/2016/03/10/good-uses-for-fake-data-part-1/
http://modernstatisticalworkflow.blogspot.com/2017/04/an-easy-way-to-simulate-fake-data-from.html
https://clayford.github.io/dwir/dwr_12_generating_data.html
And there's even a fakeR
package, which suggests that this is relatively common:
https://cran.r-project.org/web/packages/fakeR/fakeR.pdf
answered Aug 5 at 11:37
mktmkt
6,2645 gold badges27 silver badges85 bronze badges
6,2645 gold badges27 silver badges85 bronze badges
add a comment |
add a comment |
$begingroup$
I use a different word depending on the manner in which I use the data. If I have found the made-up dataset lying around and have pointed my algorithm at it in a confirmatory manner, then the word "synthetic" is fine.
However, oftentimes whenever I use this type of data, I have invented the data with the specific intent of showing off the capabilities of my algorithm. In other words, I invented data for the specific purpose of getting "good results". In such circumstances, I am fond of the term "contrived" along with an explanation of my expectations for the data. This is because I don't want anyone to make the mistake of thinking that I pointed my algorithm at some arbitrary synthetic dataset I found lying around and it really worked out well. If I have cherry-picked data (to the point of actually making it up) specifically to make my algorithm work out well, I say so. This is because such results provide evidence that my algorithm can work out well, but provide only very weak evidence that one might expect the algorithm to work out well in general. The word "contrived" really sums up nicely the fact that I have chosen the data with "good results" in mind, a priori.
"does that give the impression of fraudulent data?"
No, but, it is important to be clear about the source of any dataset and your a priori expectations as the experimenter when reporting your results on any dataset. The term "fraud" explicitly includes an aspect of having covered something up or having outright lied. The #1 way to avoid commission of fraud in science is to simply be honest and forthright about the nature of your data and your expectations. In other words, if your data are fabricated and you fail to say as much in any way, and there is some kind of expectation that the data are not fabricated or, worse, you claim that the data are gathered in some non-fabricated sort of way, then that is "fraud". Don't do that thing. If you want to use some synonym for the term "fabricated" that "sounds better", such as "synthetic", nobody will fault you, but at the same time I don't think that anyone will notice the difference except for you.
A side note:
Less obvious are circumstances where one claims to have had a priori expectations that are actually post hoc explanations. This is also fraudulent analysis of data.
There is a danger of this when one chooses data specifically with the intent of "showing off" the capabilities of an algorithm, which is frequently the case with synthetic data.
To be clear about why this is the case, consider that the "normal" scientific method works something like so: 1) A population $D$ is chosen 2) A hypothesis $H$ is concieved 3) $H$ is tested against $D$ (or some sample chosen from $D$). Science doesn't have to work within this narrow definition, but this is what is called "confirmatory" analysis, and is generally considered the strongest form of evidence one can provide. Since the order of events correlates with the strength of evidence, it is important to specifically document them.
Notably, in the case of "contrived" data, the process often works more like so: 1) A hypothesis $H$ is conceived, 2) A population $D$ is chosen, 3) $H$ is tested against $D$. If you are testing an algorithm, for example, then the hypothesis that your fancy new algorithm "does a good job" might occur prior to the invention of the synthetic dataset. If this is the case, you should mention it. At the very least you should not purport that events transpired in a "confirmatory" manner, because that would lead readers to conclude that your evidence is stronger than it actually is.
There is no problem with doing this, so long as you are honest and forthright about what you have done. If you have gone through pains to create a dataset that gives "good results", do say so. As long as you let the reader know the steps that you have taken in your data analysis, they have the information necessary to effectively weigh the evidence for or against your hypotheses. When you are not honest or are not forthright, then this may give the impression that your evidence is stronger than it really is. When you are KNOWINGLY less than honest and forthright for the sake of making your evidence seem stronger than it really is, then that is, indeed, fraudulent.
In any case, this is why I prefer the term "contrived" for such datasets, along with a short explanation that they are, indeed, chosen with a hypothesis in mind. "Contrived" conveys the sense that not only did I create a synthetic dataset, but I did so with particular intentions that reflect the fact that my hypothesis was already in place before the creation of my dataset.
To illustrate by an example: You create an algorithm for analysis of arbitrary time-series. You hypothesize that this algorithm will give "good results" when pointed at time-series. Consider, now, the following two possibilities:
1) You create some synthetic data that looks like the sort of thing that you expect your algorithm to perform well on. You analyze this data and the algorithm performs well. 2) You grab some synthetic datasets because they are available why not. You analyze this data and the algorithm performs well. Which of these two circumstances provides the better evidence that your algorithm performs well on arbitrary time-series? Clearly, it is option 2. However, it might be easy to report in either option 1 or option 2 that "we applied algorithm $A$ to synthetic dataset $D$. Results are shown in Figure $x.y$." In the absence of any context, a reader might reasonably assume that these results are confirmatory (option 2), when, in the case of option 1, they are not. The reader has therefore, in option 1, been given the impression that the evidence is stronger than it really is.
tl;dr
Use whatever term you like, "synthetic", "contrived", "fabricated", "fictitious". However, the term that you use is insufficient to ensure that your results are not misleading. Ensure that you are clear in your report about how the data came about, including your expectations for the data and the reasons why you chose the data that you chose.
$endgroup$
add a comment |
$begingroup$
I use a different word depending on the manner in which I use the data. If I have found the made-up dataset lying around and have pointed my algorithm at it in a confirmatory manner, then the word "synthetic" is fine.
However, oftentimes whenever I use this type of data, I have invented the data with the specific intent of showing off the capabilities of my algorithm. In other words, I invented data for the specific purpose of getting "good results". In such circumstances, I am fond of the term "contrived" along with an explanation of my expectations for the data. This is because I don't want anyone to make the mistake of thinking that I pointed my algorithm at some arbitrary synthetic dataset I found lying around and it really worked out well. If I have cherry-picked data (to the point of actually making it up) specifically to make my algorithm work out well, I say so. This is because such results provide evidence that my algorithm can work out well, but provide only very weak evidence that one might expect the algorithm to work out well in general. The word "contrived" really sums up nicely the fact that I have chosen the data with "good results" in mind, a priori.
"does that give the impression of fraudulent data?"
No, but, it is important to be clear about the source of any dataset and your a priori expectations as the experimenter when reporting your results on any dataset. The term "fraud" explicitly includes an aspect of having covered something up or having outright lied. The #1 way to avoid commission of fraud in science is to simply be honest and forthright about the nature of your data and your expectations. In other words, if your data are fabricated and you fail to say as much in any way, and there is some kind of expectation that the data are not fabricated or, worse, you claim that the data are gathered in some non-fabricated sort of way, then that is "fraud". Don't do that thing. If you want to use some synonym for the term "fabricated" that "sounds better", such as "synthetic", nobody will fault you, but at the same time I don't think that anyone will notice the difference except for you.
A side note:
Less obvious are circumstances where one claims to have had a priori expectations that are actually post hoc explanations. This is also fraudulent analysis of data.
There is a danger of this when one chooses data specifically with the intent of "showing off" the capabilities of an algorithm, which is frequently the case with synthetic data.
To be clear about why this is the case, consider that the "normal" scientific method works something like so: 1) A population $D$ is chosen 2) A hypothesis $H$ is concieved 3) $H$ is tested against $D$ (or some sample chosen from $D$). Science doesn't have to work within this narrow definition, but this is what is called "confirmatory" analysis, and is generally considered the strongest form of evidence one can provide. Since the order of events correlates with the strength of evidence, it is important to specifically document them.
Notably, in the case of "contrived" data, the process often works more like so: 1) A hypothesis $H$ is conceived, 2) A population $D$ is chosen, 3) $H$ is tested against $D$. If you are testing an algorithm, for example, then the hypothesis that your fancy new algorithm "does a good job" might occur prior to the invention of the synthetic dataset. If this is the case, you should mention it. At the very least you should not purport that events transpired in a "confirmatory" manner, because that would lead readers to conclude that your evidence is stronger than it actually is.
There is no problem with doing this, so long as you are honest and forthright about what you have done. If you have gone through pains to create a dataset that gives "good results", do say so. As long as you let the reader know the steps that you have taken in your data analysis, they have the information necessary to effectively weigh the evidence for or against your hypotheses. When you are not honest or are not forthright, then this may give the impression that your evidence is stronger than it really is. When you are KNOWINGLY less than honest and forthright for the sake of making your evidence seem stronger than it really is, then that is, indeed, fraudulent.
In any case, this is why I prefer the term "contrived" for such datasets, along with a short explanation that they are, indeed, chosen with a hypothesis in mind. "Contrived" conveys the sense that not only did I create a synthetic dataset, but I did so with particular intentions that reflect the fact that my hypothesis was already in place before the creation of my dataset.
To illustrate by an example: You create an algorithm for analysis of arbitrary time-series. You hypothesize that this algorithm will give "good results" when pointed at time-series. Consider, now, the following two possibilities:
1) You create some synthetic data that looks like the sort of thing that you expect your algorithm to perform well on. You analyze this data and the algorithm performs well. 2) You grab some synthetic datasets because they are available why not. You analyze this data and the algorithm performs well. Which of these two circumstances provides the better evidence that your algorithm performs well on arbitrary time-series? Clearly, it is option 2. However, it might be easy to report in either option 1 or option 2 that "we applied algorithm $A$ to synthetic dataset $D$. Results are shown in Figure $x.y$." In the absence of any context, a reader might reasonably assume that these results are confirmatory (option 2), when, in the case of option 1, they are not. The reader has therefore, in option 1, been given the impression that the evidence is stronger than it really is.
tl;dr
Use whatever term you like, "synthetic", "contrived", "fabricated", "fictitious". However, the term that you use is insufficient to ensure that your results are not misleading. Ensure that you are clear in your report about how the data came about, including your expectations for the data and the reasons why you chose the data that you chose.
$endgroup$
add a comment |
$begingroup$
I use a different word depending on the manner in which I use the data. If I have found the made-up dataset lying around and have pointed my algorithm at it in a confirmatory manner, then the word "synthetic" is fine.
However, oftentimes whenever I use this type of data, I have invented the data with the specific intent of showing off the capabilities of my algorithm. In other words, I invented data for the specific purpose of getting "good results". In such circumstances, I am fond of the term "contrived" along with an explanation of my expectations for the data. This is because I don't want anyone to make the mistake of thinking that I pointed my algorithm at some arbitrary synthetic dataset I found lying around and it really worked out well. If I have cherry-picked data (to the point of actually making it up) specifically to make my algorithm work out well, I say so. This is because such results provide evidence that my algorithm can work out well, but provide only very weak evidence that one might expect the algorithm to work out well in general. The word "contrived" really sums up nicely the fact that I have chosen the data with "good results" in mind, a priori.
"does that give the impression of fraudulent data?"
No, but, it is important to be clear about the source of any dataset and your a priori expectations as the experimenter when reporting your results on any dataset. The term "fraud" explicitly includes an aspect of having covered something up or having outright lied. The #1 way to avoid commission of fraud in science is to simply be honest and forthright about the nature of your data and your expectations. In other words, if your data are fabricated and you fail to say as much in any way, and there is some kind of expectation that the data are not fabricated or, worse, you claim that the data are gathered in some non-fabricated sort of way, then that is "fraud". Don't do that thing. If you want to use some synonym for the term "fabricated" that "sounds better", such as "synthetic", nobody will fault you, but at the same time I don't think that anyone will notice the difference except for you.
A side note:
Less obvious are circumstances where one claims to have had a priori expectations that are actually post hoc explanations. This is also fraudulent analysis of data.
There is a danger of this when one chooses data specifically with the intent of "showing off" the capabilities of an algorithm, which is frequently the case with synthetic data.
To be clear about why this is the case, consider that the "normal" scientific method works something like so: 1) A population $D$ is chosen 2) A hypothesis $H$ is concieved 3) $H$ is tested against $D$ (or some sample chosen from $D$). Science doesn't have to work within this narrow definition, but this is what is called "confirmatory" analysis, and is generally considered the strongest form of evidence one can provide. Since the order of events correlates with the strength of evidence, it is important to specifically document them.
Notably, in the case of "contrived" data, the process often works more like so: 1) A hypothesis $H$ is conceived, 2) A population $D$ is chosen, 3) $H$ is tested against $D$. If you are testing an algorithm, for example, then the hypothesis that your fancy new algorithm "does a good job" might occur prior to the invention of the synthetic dataset. If this is the case, you should mention it. At the very least you should not purport that events transpired in a "confirmatory" manner, because that would lead readers to conclude that your evidence is stronger than it actually is.
There is no problem with doing this, so long as you are honest and forthright about what you have done. If you have gone through pains to create a dataset that gives "good results", do say so. As long as you let the reader know the steps that you have taken in your data analysis, they have the information necessary to effectively weigh the evidence for or against your hypotheses. When you are not honest or are not forthright, then this may give the impression that your evidence is stronger than it really is. When you are KNOWINGLY less than honest and forthright for the sake of making your evidence seem stronger than it really is, then that is, indeed, fraudulent.
In any case, this is why I prefer the term "contrived" for such datasets, along with a short explanation that they are, indeed, chosen with a hypothesis in mind. "Contrived" conveys the sense that not only did I create a synthetic dataset, but I did so with particular intentions that reflect the fact that my hypothesis was already in place before the creation of my dataset.
To illustrate by an example: You create an algorithm for analysis of arbitrary time-series. You hypothesize that this algorithm will give "good results" when pointed at time-series. Consider, now, the following two possibilities:
1) You create some synthetic data that looks like the sort of thing that you expect your algorithm to perform well on. You analyze this data and the algorithm performs well. 2) You grab some synthetic datasets because they are available why not. You analyze this data and the algorithm performs well. Which of these two circumstances provides the better evidence that your algorithm performs well on arbitrary time-series? Clearly, it is option 2. However, it might be easy to report in either option 1 or option 2 that "we applied algorithm $A$ to synthetic dataset $D$. Results are shown in Figure $x.y$." In the absence of any context, a reader might reasonably assume that these results are confirmatory (option 2), when, in the case of option 1, they are not. The reader has therefore, in option 1, been given the impression that the evidence is stronger than it really is.
tl;dr
Use whatever term you like, "synthetic", "contrived", "fabricated", "fictitious". However, the term that you use is insufficient to ensure that your results are not misleading. Ensure that you are clear in your report about how the data came about, including your expectations for the data and the reasons why you chose the data that you chose.
$endgroup$
I use a different word depending on the manner in which I use the data. If I have found the made-up dataset lying around and have pointed my algorithm at it in a confirmatory manner, then the word "synthetic" is fine.
However, oftentimes whenever I use this type of data, I have invented the data with the specific intent of showing off the capabilities of my algorithm. In other words, I invented data for the specific purpose of getting "good results". In such circumstances, I am fond of the term "contrived" along with an explanation of my expectations for the data. This is because I don't want anyone to make the mistake of thinking that I pointed my algorithm at some arbitrary synthetic dataset I found lying around and it really worked out well. If I have cherry-picked data (to the point of actually making it up) specifically to make my algorithm work out well, I say so. This is because such results provide evidence that my algorithm can work out well, but provide only very weak evidence that one might expect the algorithm to work out well in general. The word "contrived" really sums up nicely the fact that I have chosen the data with "good results" in mind, a priori.
"does that give the impression of fraudulent data?"
No, but, it is important to be clear about the source of any dataset and your a priori expectations as the experimenter when reporting your results on any dataset. The term "fraud" explicitly includes an aspect of having covered something up or having outright lied. The #1 way to avoid commission of fraud in science is to simply be honest and forthright about the nature of your data and your expectations. In other words, if your data are fabricated and you fail to say as much in any way, and there is some kind of expectation that the data are not fabricated or, worse, you claim that the data are gathered in some non-fabricated sort of way, then that is "fraud". Don't do that thing. If you want to use some synonym for the term "fabricated" that "sounds better", such as "synthetic", nobody will fault you, but at the same time I don't think that anyone will notice the difference except for you.
A side note:
Less obvious are circumstances where one claims to have had a priori expectations that are actually post hoc explanations. This is also fraudulent analysis of data.
There is a danger of this when one chooses data specifically with the intent of "showing off" the capabilities of an algorithm, which is frequently the case with synthetic data.
To be clear about why this is the case, consider that the "normal" scientific method works something like so: 1) A population $D$ is chosen 2) A hypothesis $H$ is concieved 3) $H$ is tested against $D$ (or some sample chosen from $D$). Science doesn't have to work within this narrow definition, but this is what is called "confirmatory" analysis, and is generally considered the strongest form of evidence one can provide. Since the order of events correlates with the strength of evidence, it is important to specifically document them.
Notably, in the case of "contrived" data, the process often works more like so: 1) A hypothesis $H$ is conceived, 2) A population $D$ is chosen, 3) $H$ is tested against $D$. If you are testing an algorithm, for example, then the hypothesis that your fancy new algorithm "does a good job" might occur prior to the invention of the synthetic dataset. If this is the case, you should mention it. At the very least you should not purport that events transpired in a "confirmatory" manner, because that would lead readers to conclude that your evidence is stronger than it actually is.
There is no problem with doing this, so long as you are honest and forthright about what you have done. If you have gone through pains to create a dataset that gives "good results", do say so. As long as you let the reader know the steps that you have taken in your data analysis, they have the information necessary to effectively weigh the evidence for or against your hypotheses. When you are not honest or are not forthright, then this may give the impression that your evidence is stronger than it really is. When you are KNOWINGLY less than honest and forthright for the sake of making your evidence seem stronger than it really is, then that is, indeed, fraudulent.
In any case, this is why I prefer the term "contrived" for such datasets, along with a short explanation that they are, indeed, chosen with a hypothesis in mind. "Contrived" conveys the sense that not only did I create a synthetic dataset, but I did so with particular intentions that reflect the fact that my hypothesis was already in place before the creation of my dataset.
To illustrate by an example: You create an algorithm for analysis of arbitrary time-series. You hypothesize that this algorithm will give "good results" when pointed at time-series. Consider, now, the following two possibilities:
1) You create some synthetic data that looks like the sort of thing that you expect your algorithm to perform well on. You analyze this data and the algorithm performs well. 2) You grab some synthetic datasets because they are available why not. You analyze this data and the algorithm performs well. Which of these two circumstances provides the better evidence that your algorithm performs well on arbitrary time-series? Clearly, it is option 2. However, it might be easy to report in either option 1 or option 2 that "we applied algorithm $A$ to synthetic dataset $D$. Results are shown in Figure $x.y$." In the absence of any context, a reader might reasonably assume that these results are confirmatory (option 2), when, in the case of option 1, they are not. The reader has therefore, in option 1, been given the impression that the evidence is stronger than it really is.
tl;dr
Use whatever term you like, "synthetic", "contrived", "fabricated", "fictitious". However, the term that you use is insufficient to ensure that your results are not misleading. Ensure that you are clear in your report about how the data came about, including your expectations for the data and the reasons why you chose the data that you chose.
answered Aug 5 at 11:43


ScottScott
3981 silver badge14 bronze badges
3981 silver badge14 bronze badges
add a comment |
add a comment |
$begingroup$
Intuitively I would go to the term 'Dummy data', in the same sense that "Lorem ipsum..." is called 'Dummy text'.
The word 'Dummy' is quite general and easy to understand for people from various backgrounds and is therfore less likely to be misinterpreted by readers of a less statistical background.
$endgroup$
2
$begingroup$
If it's in a regression context, I would avoid overloading "dummy", lest you have dummy variables encoding dummy data.
$endgroup$
– Matt Krause
Aug 5 at 21:24
add a comment |
$begingroup$
Intuitively I would go to the term 'Dummy data', in the same sense that "Lorem ipsum..." is called 'Dummy text'.
The word 'Dummy' is quite general and easy to understand for people from various backgrounds and is therfore less likely to be misinterpreted by readers of a less statistical background.
$endgroup$
2
$begingroup$
If it's in a regression context, I would avoid overloading "dummy", lest you have dummy variables encoding dummy data.
$endgroup$
– Matt Krause
Aug 5 at 21:24
add a comment |
$begingroup$
Intuitively I would go to the term 'Dummy data', in the same sense that "Lorem ipsum..." is called 'Dummy text'.
The word 'Dummy' is quite general and easy to understand for people from various backgrounds and is therfore less likely to be misinterpreted by readers of a less statistical background.
$endgroup$
Intuitively I would go to the term 'Dummy data', in the same sense that "Lorem ipsum..." is called 'Dummy text'.
The word 'Dummy' is quite general and easy to understand for people from various backgrounds and is therfore less likely to be misinterpreted by readers of a less statistical background.
answered Aug 5 at 11:51
MathijsMathijs
266 bronze badges
266 bronze badges
2
$begingroup$
If it's in a regression context, I would avoid overloading "dummy", lest you have dummy variables encoding dummy data.
$endgroup$
– Matt Krause
Aug 5 at 21:24
add a comment |
2
$begingroup$
If it's in a regression context, I would avoid overloading "dummy", lest you have dummy variables encoding dummy data.
$endgroup$
– Matt Krause
Aug 5 at 21:24
2
2
$begingroup$
If it's in a regression context, I would avoid overloading "dummy", lest you have dummy variables encoding dummy data.
$endgroup$
– Matt Krause
Aug 5 at 21:24
$begingroup$
If it's in a regression context, I would avoid overloading "dummy", lest you have dummy variables encoding dummy data.
$endgroup$
– Matt Krause
Aug 5 at 21:24
add a comment |
$begingroup$
In business you would call it synthetic data predominantly - and occasionally simulated data or fake data. In academia you would call it pseudo-data predominantly, and occasionally simulated data. If it is the result of a Monte Carlo simulation, at times it gets referred colloquially as simply “Monte Carlo”.
$endgroup$
2
$begingroup$
"Monte Carlo" is the name of the method, so the "colloquial" name would be very misleading.
$endgroup$
– Tim♦
Aug 5 at 21:30
$begingroup$
It’s jargon - and as any other technical jargon is meant to create a community around it, by leaving the rest of the world wondering what the hell is this about. So yes it is used and yes it is (quite voluntarily) misleading
$endgroup$
– famargar
Aug 5 at 21:38
add a comment |
$begingroup$
In business you would call it synthetic data predominantly - and occasionally simulated data or fake data. In academia you would call it pseudo-data predominantly, and occasionally simulated data. If it is the result of a Monte Carlo simulation, at times it gets referred colloquially as simply “Monte Carlo”.
$endgroup$
2
$begingroup$
"Monte Carlo" is the name of the method, so the "colloquial" name would be very misleading.
$endgroup$
– Tim♦
Aug 5 at 21:30
$begingroup$
It’s jargon - and as any other technical jargon is meant to create a community around it, by leaving the rest of the world wondering what the hell is this about. So yes it is used and yes it is (quite voluntarily) misleading
$endgroup$
– famargar
Aug 5 at 21:38
add a comment |
$begingroup$
In business you would call it synthetic data predominantly - and occasionally simulated data or fake data. In academia you would call it pseudo-data predominantly, and occasionally simulated data. If it is the result of a Monte Carlo simulation, at times it gets referred colloquially as simply “Monte Carlo”.
$endgroup$
In business you would call it synthetic data predominantly - and occasionally simulated data or fake data. In academia you would call it pseudo-data predominantly, and occasionally simulated data. If it is the result of a Monte Carlo simulation, at times it gets referred colloquially as simply “Monte Carlo”.
answered Aug 5 at 21:06
famargarfamargar
5221 gold badge4 silver badges18 bronze badges
5221 gold badge4 silver badges18 bronze badges
2
$begingroup$
"Monte Carlo" is the name of the method, so the "colloquial" name would be very misleading.
$endgroup$
– Tim♦
Aug 5 at 21:30
$begingroup$
It’s jargon - and as any other technical jargon is meant to create a community around it, by leaving the rest of the world wondering what the hell is this about. So yes it is used and yes it is (quite voluntarily) misleading
$endgroup$
– famargar
Aug 5 at 21:38
add a comment |
2
$begingroup$
"Monte Carlo" is the name of the method, so the "colloquial" name would be very misleading.
$endgroup$
– Tim♦
Aug 5 at 21:30
$begingroup$
It’s jargon - and as any other technical jargon is meant to create a community around it, by leaving the rest of the world wondering what the hell is this about. So yes it is used and yes it is (quite voluntarily) misleading
$endgroup$
– famargar
Aug 5 at 21:38
2
2
$begingroup$
"Monte Carlo" is the name of the method, so the "colloquial" name would be very misleading.
$endgroup$
– Tim♦
Aug 5 at 21:30
$begingroup$
"Monte Carlo" is the name of the method, so the "colloquial" name would be very misleading.
$endgroup$
– Tim♦
Aug 5 at 21:30
$begingroup$
It’s jargon - and as any other technical jargon is meant to create a community around it, by leaving the rest of the world wondering what the hell is this about. So yes it is used and yes it is (quite voluntarily) misleading
$endgroup$
– famargar
Aug 5 at 21:38
$begingroup$
It’s jargon - and as any other technical jargon is meant to create a community around it, by leaving the rest of the world wondering what the hell is this about. So yes it is used and yes it is (quite voluntarily) misleading
$endgroup$
– famargar
Aug 5 at 21:38
add a comment |
Thanks for contributing an answer to Cross Validated!
- Please be sure to answer the question. Provide details and share your research!
But avoid …
- Asking for help, clarification, or responding to other answers.
- Making statements based on opinion; back them up with references or personal experience.
Use MathJax to format equations. MathJax reference.
To learn more, see our tips on writing great answers.
Sign up or log in
StackExchange.ready(function ()
StackExchange.helpers.onClickDraftSave('#login-link');
);
Sign up using Google
Sign up using Facebook
Sign up using Email and Password
Post as a guest
Required, but never shown
StackExchange.ready(
function ()
StackExchange.openid.initPostLogin('.new-post-login', 'https%3a%2f%2fstats.stackexchange.com%2fquestions%2f420525%2fhow-to-call-made-up-data%23new-answer', 'question_page');
);
Post as a guest
Required, but never shown
Sign up or log in
StackExchange.ready(function ()
StackExchange.helpers.onClickDraftSave('#login-link');
);
Sign up using Google
Sign up using Facebook
Sign up using Email and Password
Post as a guest
Required, but never shown
Sign up or log in
StackExchange.ready(function ()
StackExchange.helpers.onClickDraftSave('#login-link');
);
Sign up using Google
Sign up using Facebook
Sign up using Email and Password
Post as a guest
Required, but never shown
Sign up or log in
StackExchange.ready(function ()
StackExchange.helpers.onClickDraftSave('#login-link');
);
Sign up using Google
Sign up using Facebook
Sign up using Email and Password
Sign up using Google
Sign up using Facebook
Sign up using Email and Password
Post as a guest
Required, but never shown
Required, but never shown
Required, but never shown
Required, but never shown
Required, but never shown
Required, but never shown
Required, but never shown
Required, but never shown
Required, but never shown
sSIP1xl7uzPUTde4AFXtofD,Q51DWYbcE2SE9wWXvk88 1pzP8wuKHWSdPzlBGAPRnvVFDdvt,e68vG6zOM7Jt
8
$begingroup$
Just to add a comment which spreads across several answer: "synthetic" is a good word for made up data which tries to look as realistic as possible, while "mock up" suggests data which has been crafted to demonstrate something particular. For example, "mock up" data might contain absurd outliers, just to demonstrate how important it is to deal with outliers properly.
$endgroup$
– Cort Ammon
Aug 4 at 20:02